What is Data Annotation and Why Does Your Business Need It?
Last Updated on: April 29, 2024
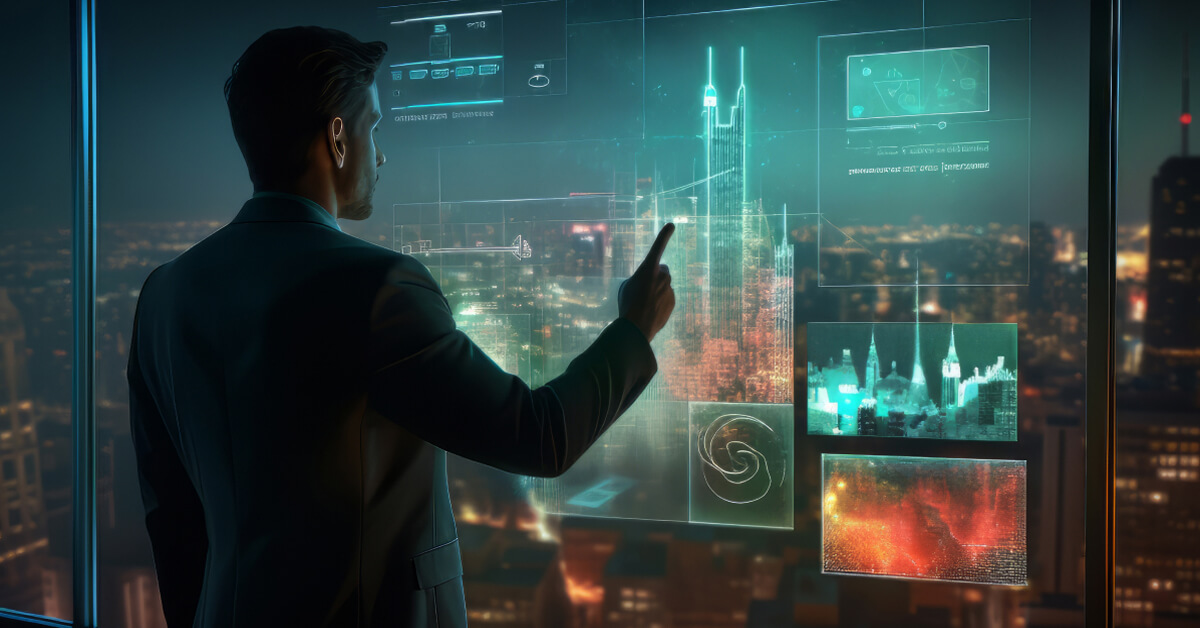
In the age of intelligent automation, artificial intelligence (AI) has become the driving force behind innovation across every industry. From self-driving cars to personalised recommendations, AI is revolutionising how we live, work, and interact with the world around us. However, the true potential of AI remains locked within the confines of unlabeled data. This blog is your comprehensive guide to data annotation.
We’ll delve into the what, why, and how of this essential process, exploring its diverse applications across various industries. We’ll also shed light on advanced techniques, emerging trends and types of data annotation, equipping you with the knowledge needed to harness the full potential of AI in your organisation.
Whether you’re a C-level executive, a business leader, or a decision-maker looking to leverage AI for your company’s success, this blog is your essential resource.
Table of Contents
Why is Data Annotation Important?
Industry-Specific Applications of Data Annotation
Advanced Techniques of Data Annotation
Emerging Trends in Data Annotation
Key Benefits of Outsourcing Data Annotation
How Systango Can Help with Data Annotation?
I. What is Data Annotation?
Imagine a child learning a new language. They need labelled examples to understand the meaning of words and sentences. Similarly,
- Data annotation acts as the teacher for AI models.
- It involves adding labels or tags to raw data, such as images, text, or audio, to provide context and meaning.
- This allows AI algorithms to accurately interpret and learn from the data, ultimately enabling them to perform specific tasks.
For instance, within the domain of self-driving cars –
- Data annotation involves labelling images and videos with information like pedestrians, traffic lights, and lane markings.
- This annotated data is then used to train AI models to recognise these objects and make critical driving decisions in real-time.
II. Why is Data Annotation Important?
High-quality annotation is the bedrock of reliable and accurate AI models. Here’s why it’s crucial for your business:
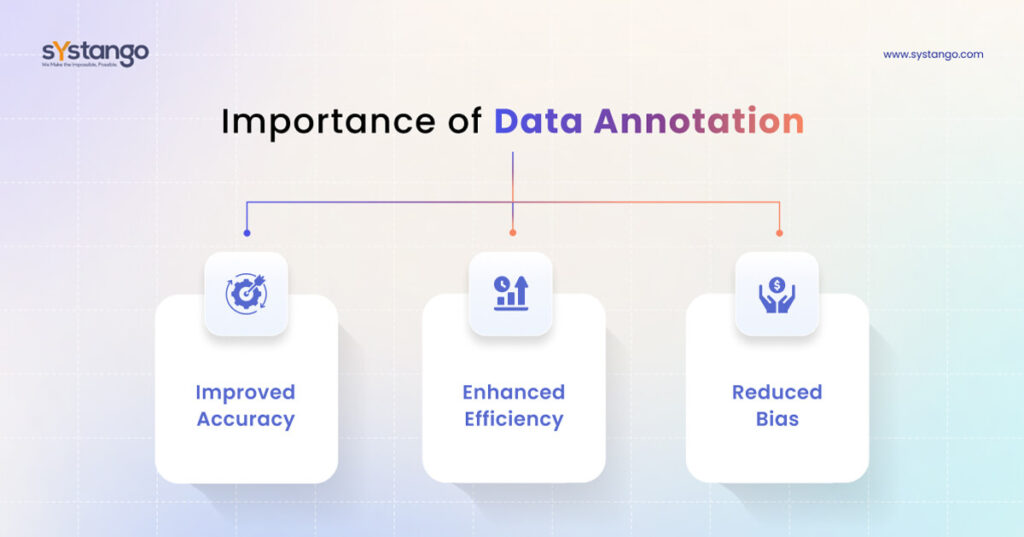
1. Improved Accuracy
- Precisely labelled data allows AI models to learn and make predictions with greater accuracy.
- This is vital for applications like medical diagnosis, fraud detection, and product recommendations, where even minor errors can have significant consequences.
Example:
Imagine an AI system analysing medical scans for tumours. If the training data isn’t meticulously annotated by medical professionals, the model might misdiagnose harmless tissues as cancerous, leading to unnecessary patient anxiety and treatment.
2. Enhanced Efficiency
- Without clear labels, AI models struggle to identify patterns and trends in data, leading to slower processing times and inefficiencies.
- Data annotation streamlines this process, enabling models to work faster and deliver results promptly.
Example:
Consider an e-commerce platform that utilises AI for product recommendations. Well-annotated data allows the model to grasp user preferences and buying habits quickly, leading to more relevant suggestions and a more efficient shopping experience for customers.
Seeking Reliable Data Annotation Solutions?
- Discover how Systango’s data annotation services can fast-track your AI development, customised to your unique needs.
3. Reduced Bias
- Unbiased data annotation practices help mitigate algorithmic bias, ensuring that AI models are fair and equitable in their decision-making.
- This is essential for maintaining ethical considerations and building trust with your customers.
Example:
Consider a bank that utilises AI for loan approvals needs to ensure its training data doesn’t contain biases based on race, gender, or socioeconomic background. Responsible annotation practices ensure the model evaluates loan applications based on creditworthiness, not demographics.
III. How is Data Annotation Done?
It can be a complex and meticulous process, often requiring human expertise. Here’s a breakdown of the typical workflow:
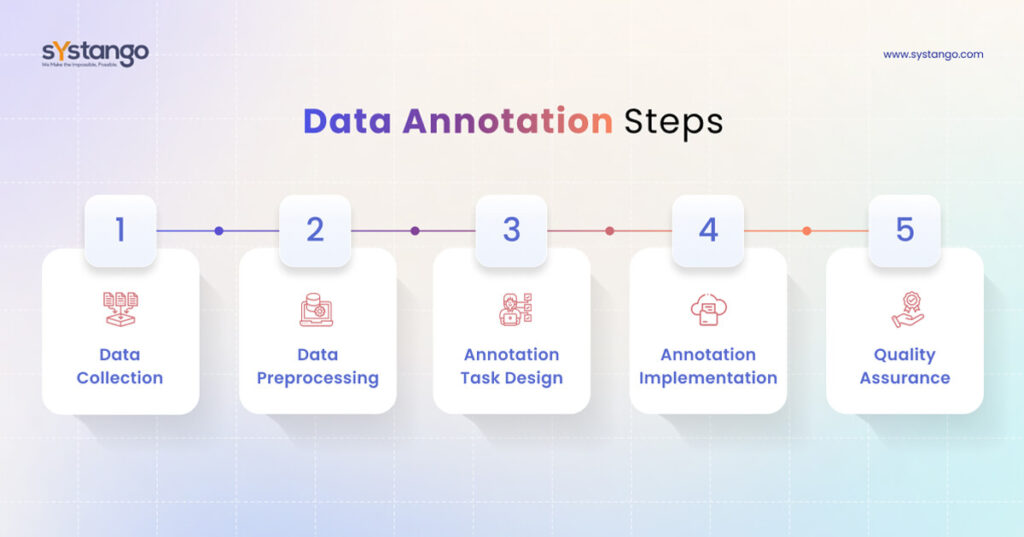
1. Data Collection
- The first step involves gathering the raw data that will be used to train the AI model.
- This data can come from various sources, including internal databases, customer surveys, sensor readings, or publicly available datasets.
2. Data Preprocessing
- The raw data might need cleaning and formatting before annotation.
- This could involve removing duplicates, correcting errors, and ensuring consistency in the data format.
3. Annotation Task Design
- Data scientists define the specific task for the annotators.
- This includes determining the type of labels required (e.g., bounding boxes for objects in images, sentiment classification for text) and establishing clear guidelines for consistent annotation.
4. Data Annotation
- The actual labelling process is often performed by human annotators, who work on specialised platforms designed for efficient and accurate annotation.
- Depending on the complexity of the task, automation tools like active learning can be used to assist human annotators.
5. Quality Assurance
- After annotation, the data undergoes a rigorous quality assurance (QA) process to ensure accuracy and consistency.
- This might involve multiple rounds of review and potential retraining of annotators if discrepancies are found.
IV. Types of Data Annotation
The specific type of annotation used depends on the data type and the AI model’s intended application. Here are the types:
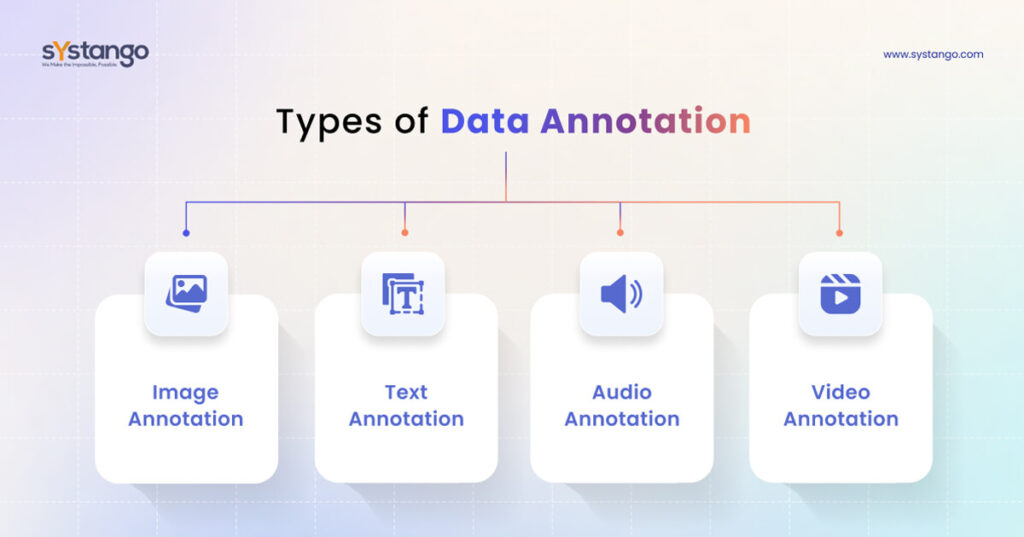
1. Image Annotation
It is essential for training AI models to interpret visual data accurately.
This process involves labelling objects, scenes, or activities within images.
- Bounding Boxes: These describe specific objects in images, vital for tasks like obstacle avoidance in self-driving cars.
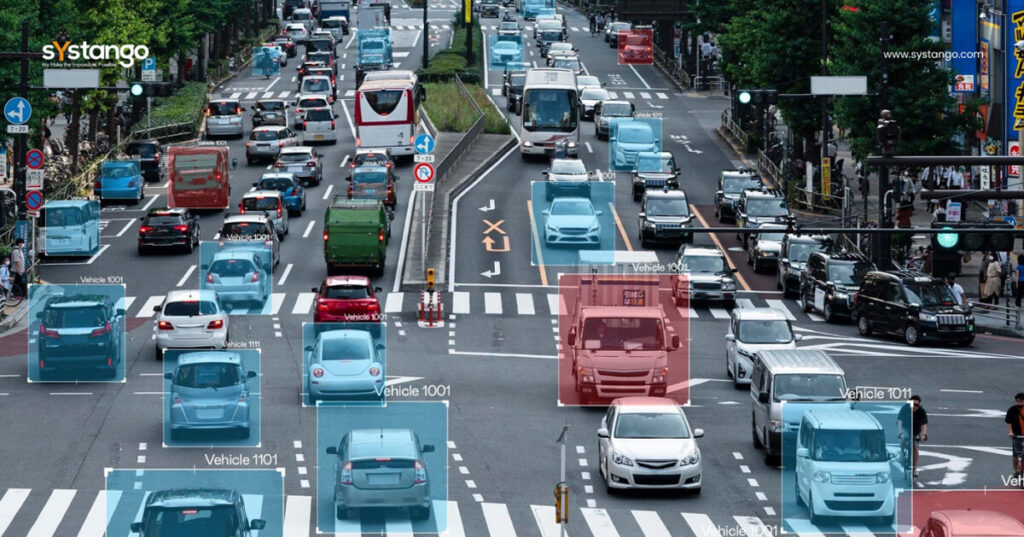
- Facial Landmark Detection: It identifies key points on faces for precise facial recognition.
- Image Segmentation: This technique partitions images for detailed analysis, particularly useful in medical imaging for identifying anatomical structures or pathological features.
2. Text Annotation
Text annotation is vital for enhancing raw text to facilitate AI comprehension by assigning labels or categories. Through text annotation, AI models gain the ability to decipher and interpret the intricate nuances embedded within textual data, enabling a wide array of applications across diverse domains.
This process includes several techniques:
- Sentiment Analysis: This method evaluates emotional tones like positive, negative, or neutral in customer reviews or social media posts.
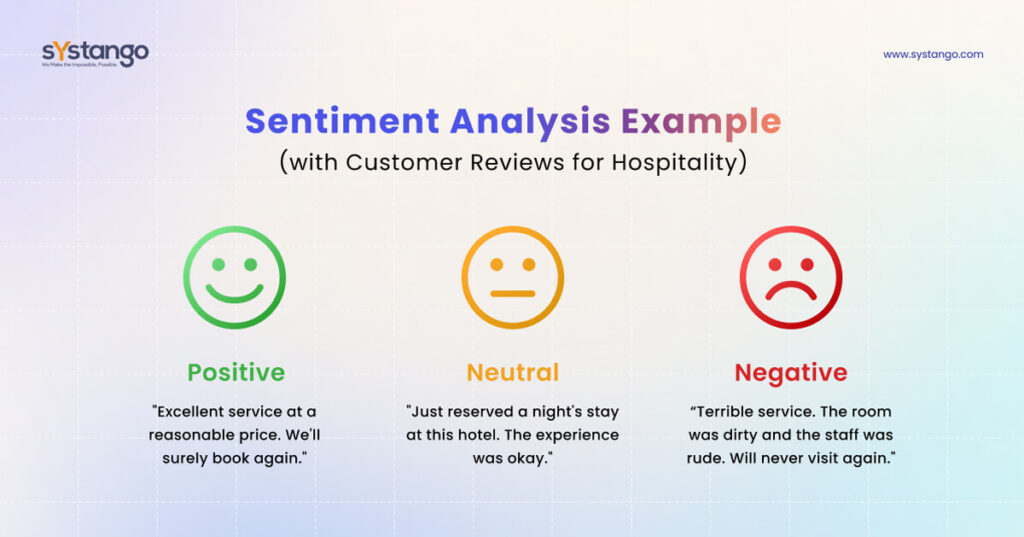
- Topic Classification: It organises the text into subjects for news categorisation or spam filtering, crucial for efficient data management.
- Named Entity Recognition (NER): NER identifies entities such as people, organisations, or locations, aiding tasks like extracting locations from emails or identifying names in medical reports.
3. Audio Annotation
Audio annotation is integral for training AI models to comprehend and process audio data effectively. This process entails labelling audio data with various types of information, enabling machines to understand and analyse sound-based information accurately.
Below are the various techniques involved in this process:
- Speaker Identification: Distinguishing between speakers in recordings enables tasks like speaker segmentation and voice profiling.
- Speech Recognition: Transcribing spoken words into text facilitates applications like virtual assistants and automated transcription services.
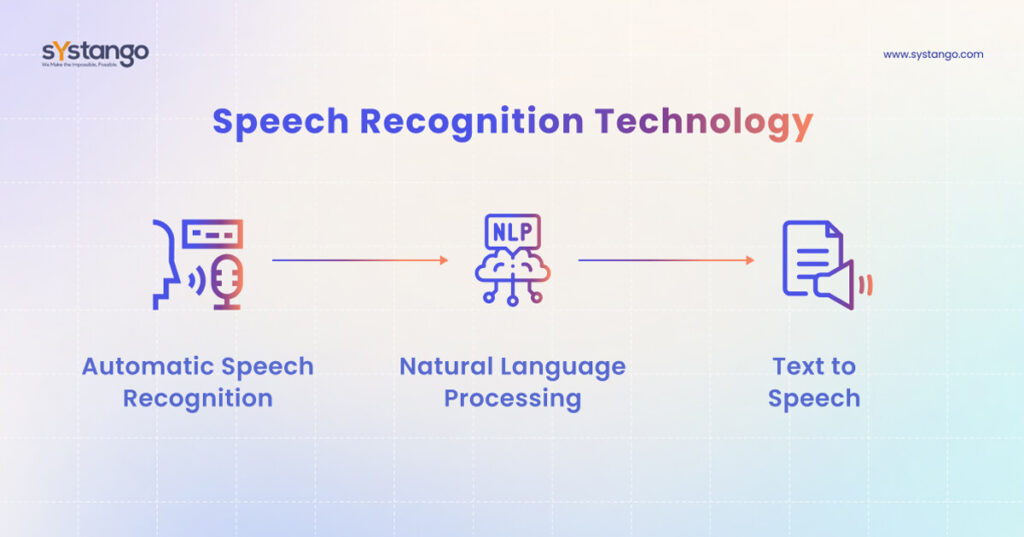
- Sound Event Detection: Identifying specific sounds such as gunshots or breaking glass enables applications like gunshot detection systems and environmental monitoring.
4. Video Annotation
Video annotation merges elements from image and audio annotation by labelling objects, actions, or events within video data. This process is vital for training AI models and supporting tasks like video surveillance, sports analytics, and autonomous drone operations.
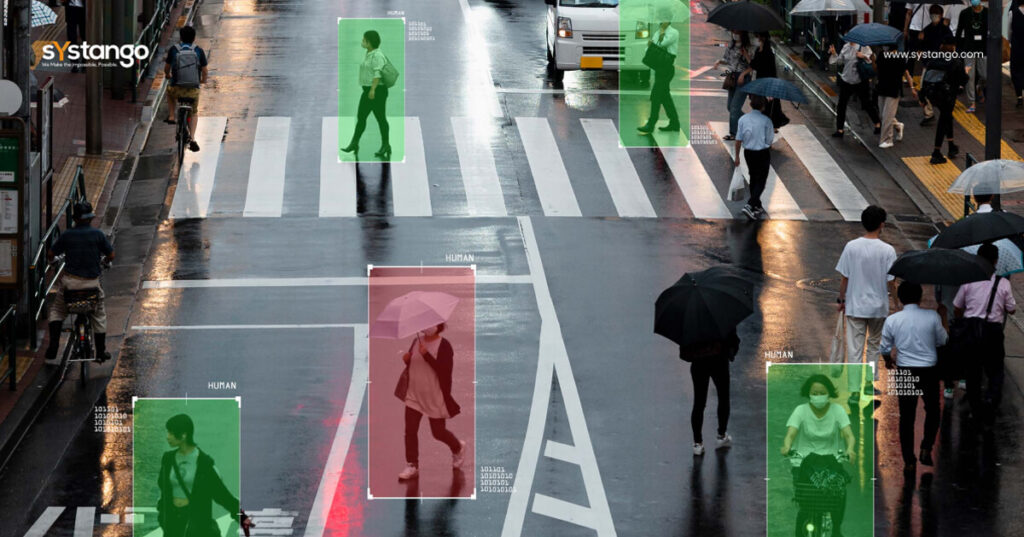
This process encompasses the following techniques:
- Object Labelling: Identifying objects in video frames aids in object detection and tracking for surveillance.
- Action Recognition: Labelling human actions enables accurate analysis of dynamic scenes.
- Event Detection: Recognising specific events allows AI systems to respond effectively, such as detecting emergencies in real-time.
V. Industry-Specific Applications of Data Annotation
Data annotation serves a critical role across various industries, empowering AI to perform groundbreaking tasks and driving innovation at an unprecedented pace.
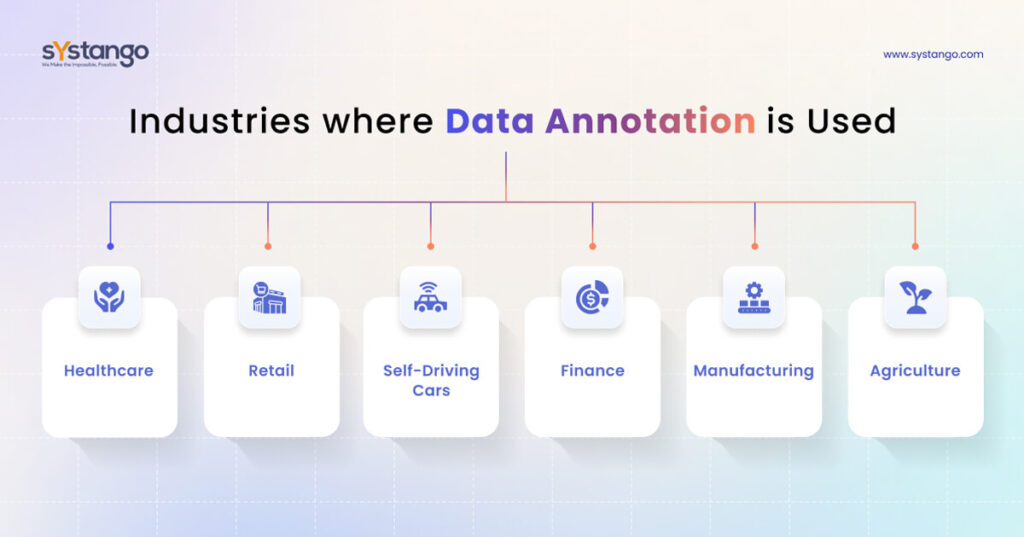
1. Healthcare
Meticulously labelled medical images train AI models to detect diseases with remarkable accuracy, leading to earlier diagnoses and improved patient outcomes.
2. Retail
It empowers AI to personalise product recommendations for customers based on their labelled preferences and purchase history. Imagine a world where your online shopping experience feels like having a personal shopping assistant who remembers your favourite brands and styles.
3. Self-Driving Cars
Annotated data with information like pedestrians, traffic lights, and lane markings plays a vital role in training AI models to navigate the complexities of the road. This ensures self-driving cars can make critical driving decisions in real-time, paving the way for a safer and more convenient transportation future.
Struggling with slow response time of in-house data annotation?
- Systango’s global workforce and efficient workflows can deliver high-quality training data faster and at a lower cost.
These are just a few examples, and the potential applications of data annotation extend far and wide, impacting industries like:
4. Finance
Data annotation plays a crucial role in identifying fraudulent transactions, safeguarding financial systems, and expediting loan approvals to ensure the smooth functioning of financial operations.
5. Manufacturing
Here, predictive analytics for equipment failures enable preventive maintenance strategies that minimise downtime, enhance overall production efficiency, and boost profitability.
6. Agriculture
It facilitates the analysis of weather patterns and soil conditions, empowering farmers to optimise crop yields, promote sustainable farming practices, and contribute to the resilience and efficiency of the global food supply chain.
Our Reading Recommendation: AI for Data Analytics: The Ultimate Guide to Boosting Insights and Action
VI. Advanced Techniques of Data Annotation
Data annotation has evolved beyond solely relying on manual processes and now combines manual and automated processes for improved effectiveness. Advanced techniques are emerging to enhance accuracy, speed, and scalability:
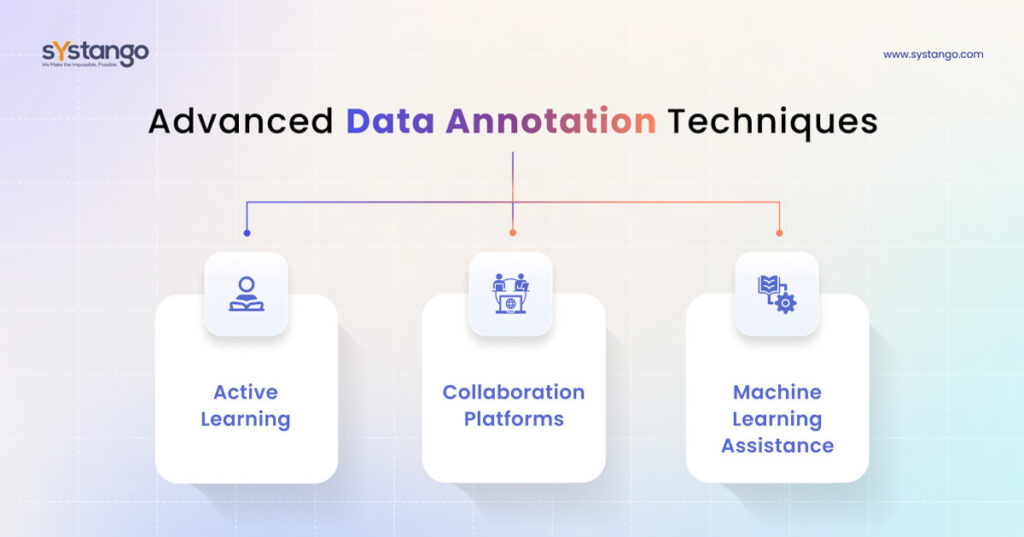
1. Active Learning
Imagine a student focusing on the most challenging concepts first to solidify their understanding. Similarly, active learning prioritises data points that maximise the learning efficiency of AI models, allowing annotators to focus their efforts on the most impactful tasks.
2. Collaboration Platforms
These platforms enable teams of annotators to work together on complex projects. This streamlines the workflow, ensures consistency in labelling practices, and fosters a collaborative environment for knowledge sharing.
3. Machine Learning Assistance
Machine learning algorithms can pre-label data based on existing patterns, freeing up human annotators to focus on complex or ambiguous cases. This collaborative approach between human expertise and machine learning augments the overall process, leading to a more efficient and accurate data annotation experience.
VII. Emerging Trends in Data Annotation
Data annotation is a rapidly evolving field with continuous advancements in technology and techniques. Here are some exciting trends to watch:
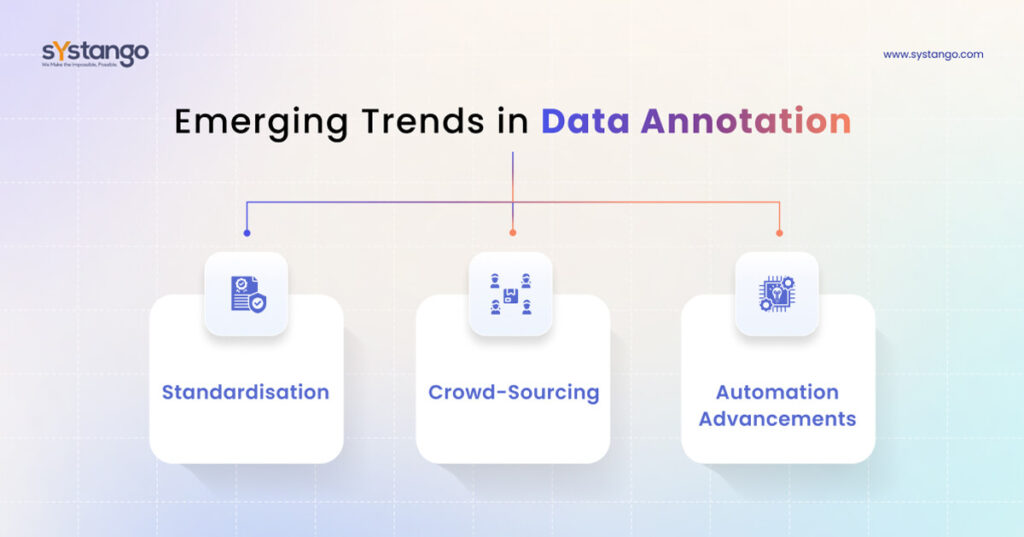
1. Standardisation
Efforts are underway to create consistent annotation formats across different platforms. This fosters better interoperability and reduces complexity, making data annotation a more seamless process.
2. Crowd-Sourcing
Crowd-sourcing platforms are emerging as cost-effective solutions for tasks suited to a broader workforce. This allows for faster completion of large-scale projects, particularly for tasks that don’t require specialised knowledge.
3. Automation Advancements
Machine learning models will play an even larger role in data pre-labelling and quality control, further streamlining the annotation process. This frees up human annotators for tasks requiring higher-level judgement and ensures consistent quality throughout the data set.
These trends point towards a future where annotation is not only faster and more efficient but also empowers the development of even more powerful AI applications across all industries.
VIII. Key Benefits of Outsourcing Data Annotation
While it can be done in-house, many businesses choose to outsource this task to specialised data annotation companies. Here are some key benefits of outsourcing:
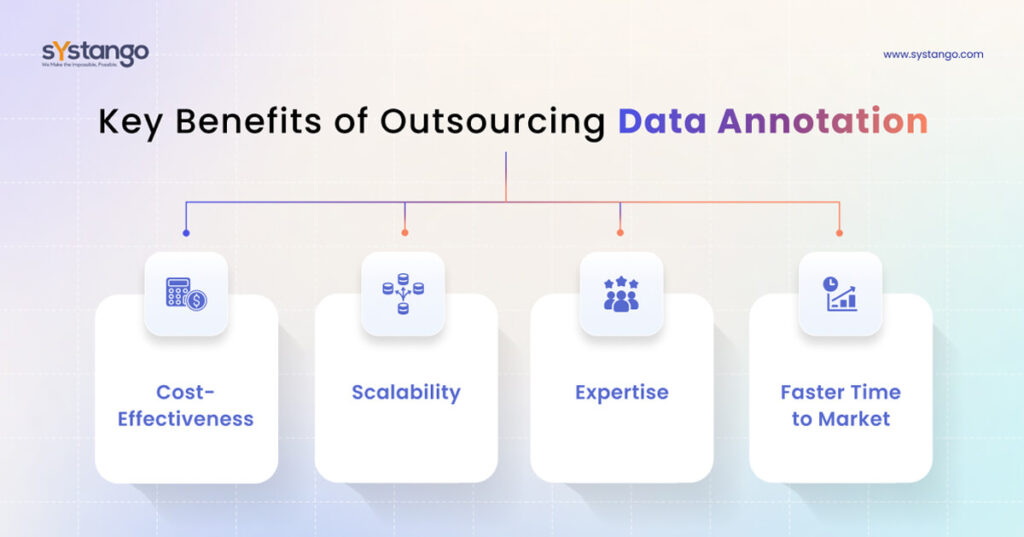
1. Cost-Effectiveness
Data annotation can be a labour-intensive process. Outsourcing allows you to leverage a global workforce with competitive rates, potentially leading to significant cost savings.
2. Scalability
This type of projects can require many annotators, especially for big data applications. Outsourcing partners have the infrastructure and workforce to scale their operations up or down based on your project needs.
3. Expertise
Data annotation companies employ experienced and well-trained annotators familiar with various annotation types and best practices. This ensures the quality and consistency of your annotated data.
4. Faster Time to Market
Outsourcing this process can significantly reduce the time it takes to train your AI models and get them deployed into production. This allows you to capitalise on the benefits of AI sooner.
IX. How Systango Can Help with Data Annotation?
Systango understands the importance of high-quality annotation for reliable AI models. Our experienced team tackles complex projects with a focus on:
- Accuracy & Efficiency: We leverage advanced tools and methodologies to ensure high-quality labelled data is delivered efficiently.
- Scalability & Cost-Effectiveness: Our global workforce of trained annotators provides cost-competitive solutions for projects of any size.
- Customisation: We tailor our approach to your needs, from image annotation to sentiment analysis.
- Quality Guaranteed: Robust QA procedures ensure the consistency and integrity of your data.
By partnering with Systango, you gain access to data annotation experts who can streamline your AI development process and empower you to unlock the full potential of machine learning. Contact us today to discuss your requirements.
You might also like to read: Demystifying Explainable AI: Lifting the Lid on AI’s Thinking
Concluding Thoughts
Data annotation, the foundation of robust AI models, plays a critical role in unlocking the true potential of artificial intelligence.
- Through meticulous labelling of raw data, it empowers AI to understand and interpret the world, fuelling groundbreaking applications across diverse industries.
- Advancements in standardisation, crowd-sourcing, and automation promise a more efficient and streamlined approach to this process in the future.
- This paves the way for the development of even more powerful AI applications that will transform how we live, work, and interact with the world.
By embracing data annotation as a strategic investment, businesses can position themselves at the forefront of the AI revolution. Whether you’re a C-level executive, a business leader, or a decision-maker looking to leverage AI for your company’s success, we encourage you to explore the transformative potential of data annotation.
Seeking Reliable Data Annotation Solutions?
- Discover how Systango’s data annotation services can fast-track your AI development, customised to your unique needs.