Generative AI in Finance: Integration Methods, Business Applications, Regulatory Challenges and More
Last Updated on: May 6, 2025
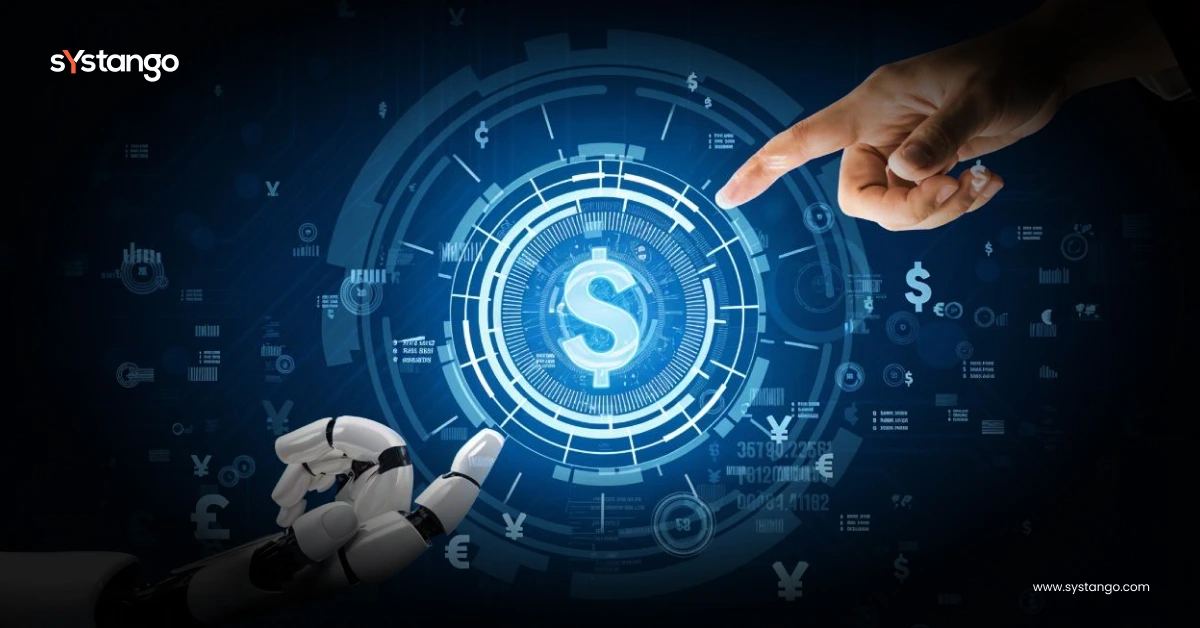
The finance industry is undergoing a transformative shift, and at the heart of this revolution is Generative AI. Business leaders are turning to AI-driven solutions to streamline operations, enhance customer experiences, and drive innovation. This blog will explore how Generative AI in Finance is reshaping financial services, offering business leaders insights on maximising efficiency, minimising risks, and leveraging AI to boost ROI. Whether you’re looking to optimise operations or stay ahead of the competition, this blog will provide valuable strategies for integrating AI into your financial systems.
Key Takeways
I. What is Generative AI in Finance?
II. Traditional AI vs Generative AI in Finance
III. Top Business Applications of Generative AI in Finance
IV. Optimising Business ROI with Generative AI in Finance
V. How Can Businesses Implement Generative AI in FinTech Systems?
VI. Regulatory Challenges and Compliance Strategies
VII. Case Studies: Real-World Use of Generative AI in FinTech
VIII. Future Trends: The Evolving Role of Generative AI in Finance
IX. Why Systango Is Your Ideal Generative AI Partner?
I. What is Generative AI in Finance?
Generative AI uses advanced algorithms to generate predictions, data, and insights based on existing information. In finance, this includes automating processes like fraud detection, financial forecasting, and personalised client recommendations.
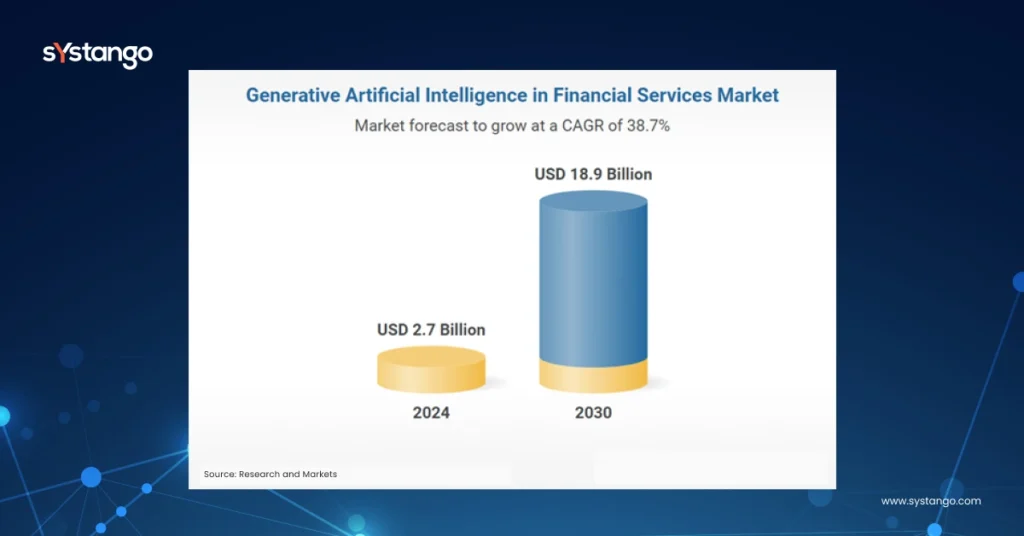
According to market research from ResearchAndMarkets, the global market for Generative AI in financial services is projected to grow from US$2.7 billion in 2024 to US$18.9 billion by 2030, at a CAGR of 38.7%.
Unlike traditional systems, Generative AI can synthesise new data and create customised solutions, offering businesses deeper insights and more proactive decision-making. This shift is not just about improving efficiency; Generative AI empowers businesses to offer more tailored and scalable services. But as its capabilities grow, the real question becomes: How does it compare to traditional AI?
In the next section, we’ll explore the key differences between traditional AI and Generative AI, and how each can benefit businesses in the financial sector.
II. Traditional AI vs Generative AI in Finance
While both Traditional AI and Generative AI are transforming the financial services industry, they differ significantly in their approaches, capabilities, and the types of insights they provide. Traditional AI focuses primarily on analysing historical data to make predictions or automate processes, typically used for fraud detection and credit scoring. In contrast, Generative AI goes a step further by not only interpreting data but also creating new content, scenarios, and synthetic datasets, offering richer insights for innovation and personalised solutions.
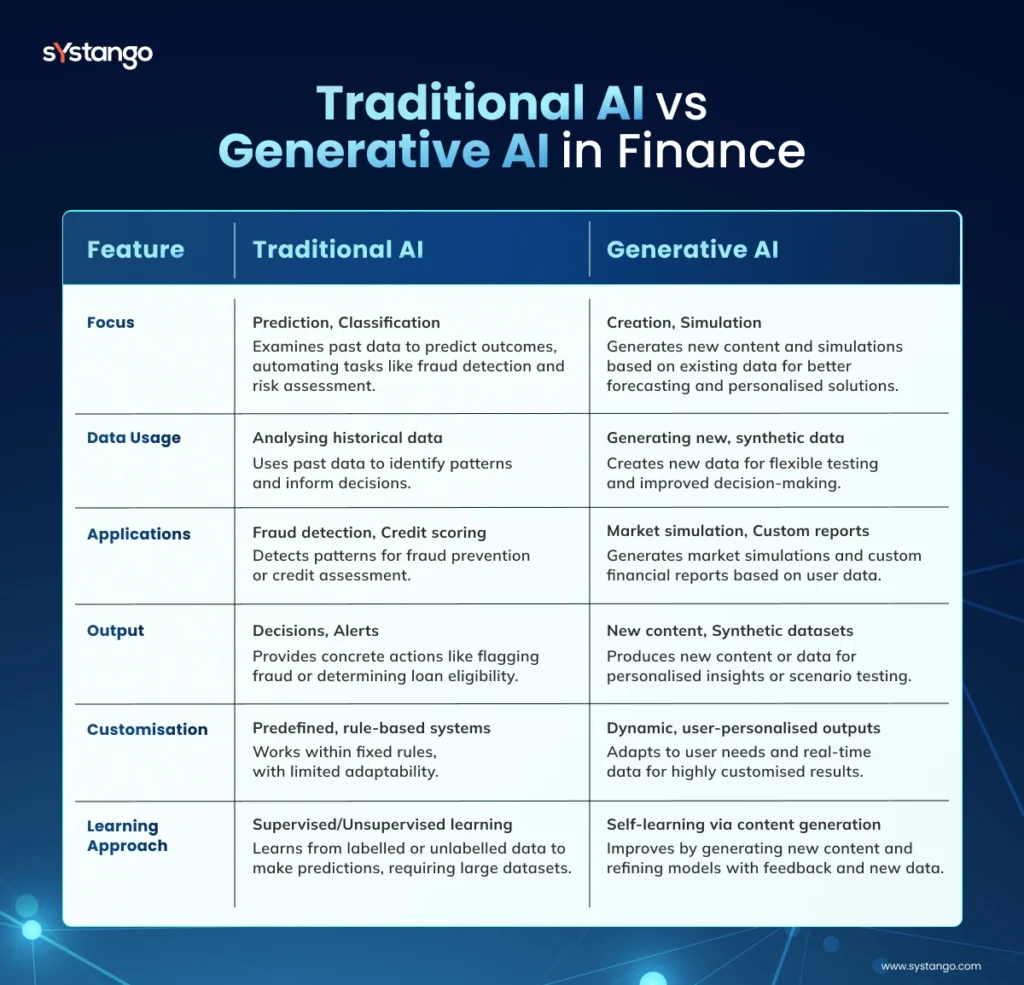
Traditional AI focuses on optimising existing processes through historical data analysis, while Generative AI introduces innovation by creating new data and scenarios that enable more flexible, personalised, and forward-thinking financial solutions.
III. Top Business Applications of Generative AI in Finance
Generative AI is transforming the financial services industry by offering innovative solutions to improve operational efficiency, customer engagement, and decision-making. Here are some of the top applications where generative AI is making a significant impact:
1. Personalised Customer Experiences
Generative AI enables financial institutions to offer hyper-personalised services to clients.
- By analysing vast amounts of customer data, AI models like GPT-4 can generate tailored financial advice, recommend personalised investment strategies, and even simulate personalised financial scenarios.
- This not only improves customer satisfaction but also enhances loyalty and retention.
2. Risk Assessment and Management
Generative AI can help financial firms manage risk by generating predictive models that simulate various risk scenarios, resulting in Fintech AI applications.
- These models analyse market trends, credit histories, and other financial data to forecast potential risks, enabling financial institutions to implement proactive measures.
- For example, AI can simulate stress tests for portfolios or generate alternative credit scoring models to predict borrower risk more accurately.
3. Fraud Detection and Prevention
Generative AI plays a crucial role in enhancing fraud detection capabilities.
- By analysing transactional data in real-time, it can generate anomaly detection models that identify suspicious activities.
- Generative models, like adversarial networks, can simulate fraudulent transactions, helping financial institutions develop systems that are better equipped to prevent fraud before it occurs.
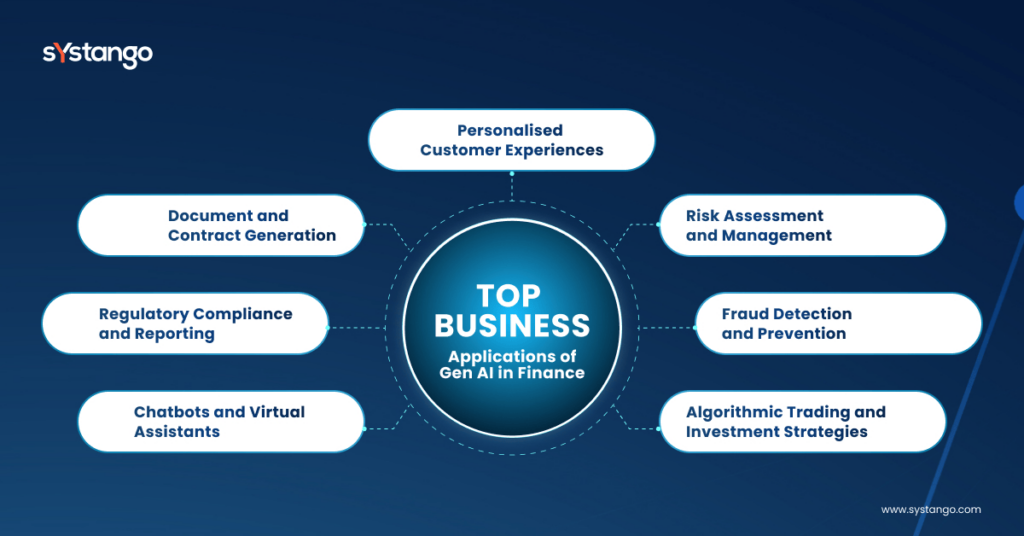
4. Algorithmic Trading and Investment Strategies
In trading, generative AI can create predictive models that simulate different market conditions and generate optimal trading strategies.
- These AI-powered models can forecast stock price movements, automate trading decisions, and adjust strategies in real-time.
- As a result, financial firms can make faster, data-driven decisions and gain a competitive edge in the market.
5. Document and Contract Generation
In financial services, document generation is an essential part of customer onboarding, contract creation, and compliance.
- Generative AI can streamline this process by automatically generating legal documents, such as contracts, loan agreements, and regulatory reports, based on predefined templates and rules.
- This reduces human error and significantly speeds up the process of document creation.
6. Regulatory Compliance and Reporting
Financial institutions are heavily regulated, and maintaining compliance is a critical task.
- Generative AI can automate the generation of compliance reports by analysing transaction data and flagging any potential non-compliance issues.
- AI systems can also simulate various regulatory scenarios, helping financial firms prepare for audits and regulatory reviews more efficiently, hence stressing the importance of Fintech AI systems.
7. Chatbots and Virtual Assistants
Generative AI is improving the capabilities of chatbots and virtual assistants in the financial industry.
- By using natural language processing (NLP), AI can generate human-like interactions, providing customers with real-time assistance on everything from account management to loan inquiries.
- These AI-driven assistants reduce the burden on customer support teams while enhancing customer service quality.
IV. Optimising Business ROI with Generative AI in Finance
Generative AI holds immense potential for financial institutions seeking to boost their return on investment (ROI). By streamlining operations, improving decision-making, and enhancing customer engagement, generative AI helps financial businesses achieve higher efficiency and profitability. Here are several ways generative AI optimises ROI in finance:
1. Automating Time-Consuming Tasks: Streamlining Operations for Maximum Efficiency
Generative AI can significantly reduce the time spent on repetitive and mundane tasks, enabling financial institutions to focus on more strategic operations. Automating routine processes—such as customer inquiries, report generation, and document handling—helps businesses achieve greater efficiency while lowering operational costs.
Key Benefits:
- Faster Execution: Tasks are completed in a fraction of the time compared to manual processes.
- Cost Savings: Reduces the need for manual labour, cutting down on operational expenses.
- Increased Efficiency: Allows teams to focus on higher-value activities, driving productivity.
By incorporating automation into day-to-day workflows, financial firms can create smoother operations and increase their overall ROI.
2. Enhanced Data-Driven Decision-Making: Turning Data into Profit
Generative AI excels in processing large volumes of data, uncovering hidden patterns and trends that help businesses make informed, data-backed decisions. Whether it’s forecasting market trends, optimising loan approvals, or assessing risks, AI models ensure that decisions are more accurate, reducing the margin for error and enhancing profitability.
Key Benefits:
- Smarter Decisions: AI generates actionable insights that inform critical business strategies.
- Accuracy: Reduces human error, resulting in more reliable predictions.
- Profitability: Better decision-making leads to optimised business strategies and higher returns.
AI’s ability to analyse and generate data-driven insights empowers financial firms to refine their strategies, resulting in better business outcomes.
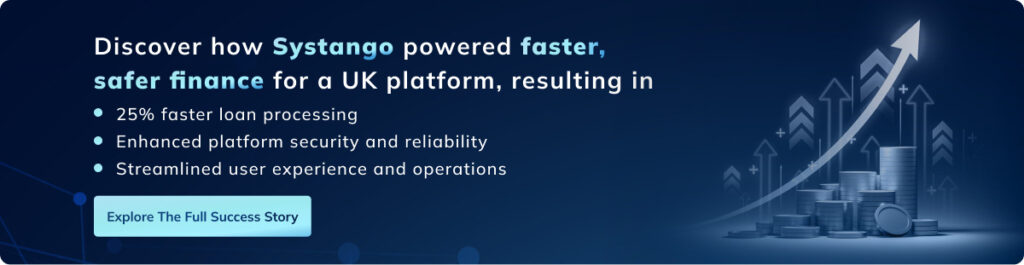
3. Improved Customer Retention and Acquisition: Personalising Every Interaction
Personalisation is crucial in today’s competitive financial market. By analysing customer data, generative AI enables financial firms to tailor their services to individual preferences. This customised approach leads to enhanced customer experiences, which not only boosts satisfaction but also drives higher retention rates and new customer acquisitions.
Key Benefits:
- Customised Financial Plans: AI generates tailored solutions based on customer behaviour and preferences.
- Targeted Marketing: AI helps design personalised marketing strategies that resonate with specific segments.
- Reduced Churn: Customers feel valued and are more likely to remain loyal to customised services.
By offering these experiences, financial institutions can maximise customer lifetime value and generate more revenue over time.
4. Optimising Asset Management and Investment Strategies: AI-Powered Portfolio Perfection
Generative AI is transforming asset management by providing financial institutions with the tools to simulate various market scenarios. AI can generate diverse investment strategies and optimise portfolios, taking into account real-time data, risk factors, and market conditions. This ensures that asset management is always aligned with the latest market trends.
Key Benefits:
- Real-Time Trading Signals: AI generates actionable insights based on current market data.
- Portfolio Optimisation: Helps businesses fine-tune their portfolios to maximise returns.
- Risk Management: AI evaluates and mitigates risks associated with investments.
By leveraging AI, financial firms can maintain dynamic and profitable investment strategies that adapt quickly to market shifts.
5. Reducing Operational Costs: Boosting Profitability Through Automation
Generative AI plays a pivotal role in driving cost efficiency. By automating functions like fraud detection, customer service, and data entry, AI reduces the reliance on manual labour, lowering operational costs and decreasing the chances of human error. This results in more efficient operations and a direct boost to profitability.
Key Benefits:
- Cost Reduction: Automates routine tasks, lowering the need for manual oversight.
- Improved Accuracy: AI minimises human errors, enhancing operational reliability.
- Scalability: AI-powered systems can handle increased workloads without a proportional rise in costs.
This level of automation leads to improved scalability and better ROI by reducing operational overhead.
6. Enhancing Risk Management: Predicting and Mitigating Financial Threats
Generative AI enhances risk management by providing predictive models that can simulate various financial scenarios, such as market fluctuations or potential fraud. These models help financial institutions identify risks early and implement proactive measures to mitigate them, safeguarding the business from potential losses.
Key Benefits:
- Proactive Risk Mitigation: AI predicts potential threats and enables early intervention.
- Cost Prevention: Minimises financial losses by foreseeing and addressing risks in advance.
- Improved Decision-Making: Provides clearer insights into potential threats, guiding risk management strategies.
By enhancing risk management capabilities, financial firms can protect themselves from costly mistakes and improve profitability.
7. Accelerating Product Development: Getting to Market Faster
Generative AI speeds up the product development cycle by analysing customer needs, market trends, and emerging demands. This helps financial institutions quickly identify new opportunities, develop products tailored to customer expectations, and reduce time-to-market, ensuring they stay ahead of competitors.
Key Benefits:
- Faster Time-to-Market: AI accelerates the development of new financial products.
- Innovative Solutions: Generates product ideas based on customer insights and market trends.
- Competitive Edge: Firms can launch new products faster, gaining an advantage over rivals.
Here’s a summarised version for a better understanding:
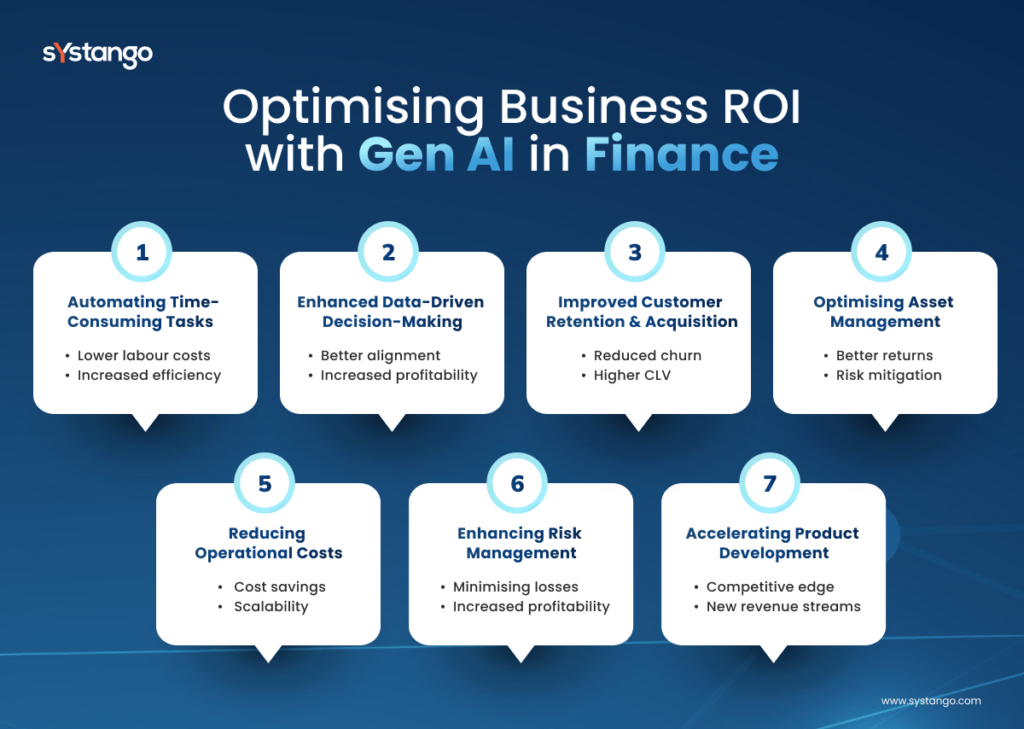
With AI driving product innovation, businesses can seize market opportunities quickly and tap into new revenue streams.
V. How Can Businesses Implement Generative AI in FinTech Systems?
Successfully implementing Generative AI in banking operations or FinTech platforms requires a strategic, phased approach—especially in an industry where compliance, risk, and data integrity are paramount. Below are the best practices for integrating AI and FinTech systems:
Step 1: Define the Use Case and ROI
Start by identifying high-value, low-risk areas—customer support, document automation, or KYC processing. Conduct a cost-benefit analysis of AI integration in financial institutions to ensure strategic alignment.
Step 2: Ensure Data Readiness
High-quality data is the lifeblood of AI Software Development. Financial institutions must invest in data cleaning, integration, and governance before any AI model can be trained effectively.
Step 3: Choose the Right Model
Decide between building in-house models or leveraging third-party Generative AI fintech platforms. The latter is faster to deploy, while custom models offer more control and specificity.
Step 4: Regulatory and Ethical Readiness
Factor in regulatory considerations for AI in finance from the outset. This includes explainability, audit trails, fairness checks, and adherence to privacy laws like GDPR and the upcoming EU AI Act.
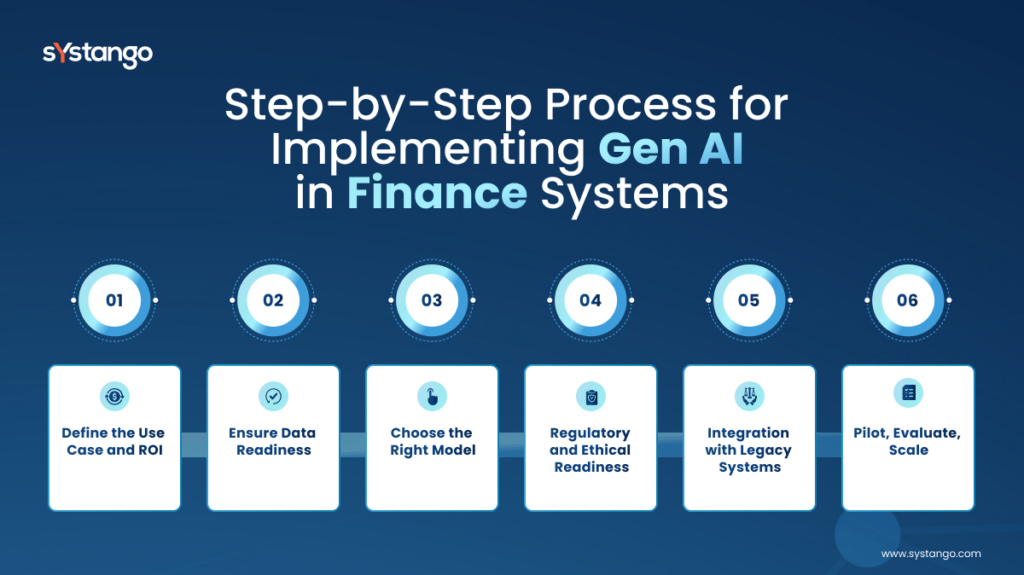
Step 5: Integration with Legacy Systems
One of the major challenges in deploying Generative AI in legacy financial systems is compatibility. APIs, modular architectures, and middleware tools can bridge new AI solutions with old infrastructure.
Step 6: Pilot, Evaluate, Scale
Run small pilots, evaluate outcomes against pre-defined KPIs, and then scale across other units. A/B testing can validate the model’s effectiveness before full-scale rollout.
VI. Regulatory Challenges and Compliance Strategies
While Generative AI in Finance offers immense innovation potential, it also brings forth regulatory and ethical complexities. Governments and financial authorities are racing to catch up with the speed of AI deployment in the sector.
The American Bankers Association states that strict AI rules and risk concerns make financial institutions cautious about adopting generative AI.
Key Regulatory Challenges
- Explainability and Transparency: Financial institutions must ensure that AI in FinTech decision-making processes are interpretable, especially in credit scoring, fraud detection, and compliance tasks.
- Bias and Fairness: Algorithms trained on biased or incomplete data may result in discriminatory lending or risk profiling, posing a reputational and legal risk.
- Data Privacy: With Generative AI fintech platforms accessing sensitive customer data, compliance with GDPR, CCPA, and similar data protection laws is non-negotiable.
- Auditability: Regulatory bodies require all AI-driven decisions, especially those involving risk and investment, to be auditable. This is challenging with black-box models.
Compliance Best Practices
- Implement a Responsible AI Framework: Establish governance protocols, explainability layers, and AI ethics boards to oversee development and deployment.
- Regular Model Audits: Conduct periodic reviews and validations of AI models to ensure consistency, accuracy, and fairness.
- Real-time Monitoring: Use tools that continuously monitor output and flag anomalies in real time.
- Collaborate with Regulators: Proactively engage with financial watchdogs to align innovations with emerging compliance standards.
By embedding regulation into design and development stages, businesses can responsibly scale AI fintech capabilities without breaching ethical or legal thresholds.
VII. Case Studies: Real-World Use of Generative AI in FinTech
Let’s take a look at how leading firms are leveraging Generative AI in FinTech to solve specific challenges and create new value streams.
Case Study 1: Morgan Stanley – AI-Powered Wealth Management
Morgan Stanley launched a GPT-4-powered assistant to support 16,000 financial advisors with real-time responses to queries about markets, internal research, and client portfolios. This marks a shift toward AI-driven personalised financial advisory services that enhance both customer experience and internal productivity.
Case Study 2: Sila – Instant Payments with API Upgrade
Sila upgraded its Money API to enhance speed and accessibility for FinTech businesses. Key updates included instant settlements, wire transfer functionality, and expanded payment options, including crypto and card processing. This upgrade improved user control and broadened platform reach.
Systango played a key role in enhancing the platform’s functionality, ensuring seamless integration and improved user engagement.
Case Study 3: HSBC – Compliance Document Automation
HSBC is leveraging AI software development to create tools that automatically generate regulatory reports and compliance checklists, streamlining operations and reducing turnaround considerably.
These case studies highlight the practical benefits of Generative AI in FinTech, driving operational efficiency, scalability, and personalised customer experiences.
VIII. Future Trends: The Evolving Role of Generative AI in Finance
The future of Generative AI in Finance is poised for rapid evolution, shaped by advancements in model architecture, regulatory clarity, and growing enterprise adoption.
1. Autonomous Finance Functions
As models become more robust, we will see increased automation in complex tasks like portfolio management, treasury operations, and even mergers and acquisitions due diligence. AI in FinTech will shift from support tools to decision-making partners.
2. Hyper-Personalised Financial Services
Generative AI Applications will drive hyper-personalised financial journeys, offering real-time nudges, dynamic budgeting, and contextual product recommendations. Expect deeper behavioural modelling powered by real-time data.
3. Embedded Finance and AI Integration
AI will increasingly be embedded into third-party platforms—retail apps, e-commerce checkouts, or insurance portals. This will expand the reach of FinTech and AI beyond traditional banking to ecosystem-based financial experiences.
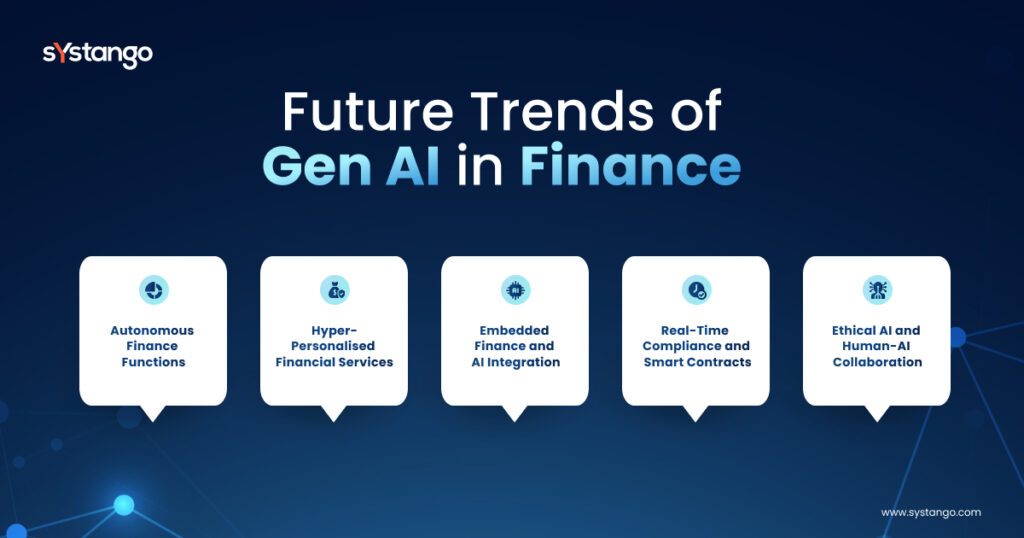
4. Real-Time Compliance and Smart Contracts
With advancements in AI Software Development, financial compliance will become proactive. AI-driven tools will continuously scan for regulatory changes and adjust policies or smart contracts in real-time, paving the way for truly intelligent regulation-as-a-service.
5. Ethical AI and Human-AI Collaboration
There will be a growing emphasis on transparent, auditable, and fair AI models. Human-in-the-loop systems will become standard, blending the cognitive power of Generative AI FinTech platforms with human judgment.
The shift is clear: finance is no longer just digital—it’s becoming cognitively intelligent.
IX. Why Systango Is Your Ideal Generative AI Partner?
At Systango, we specialise in building and integrating cutting-edge Generative AI Applications tailored specifically for the financial sector. Whether you require custom AI Software Development, sophisticated risk assessment models, automated compliance tools, or hyper-personalised client experiences, our expert team can help.
As a leading Generative AI company, we understand that in finance, innovation must go hand-in-hand with regulatory rigour, data privacy, and trust. Our services include:
- End-to-end Generative AI in FinTech consulting—from use case discovery to deployment.
- Development of secure, scalable, and auditable AI Software Development solutions.
- Fine-tuning large language models (LLMs) for specific financial tasks such as investment advisory, KYC automation, fraud detection, and reporting.
- Seamless integration of AI into existing legacy banking or FinTech infrastructure with minimal disruption.
- Ongoing support for model governance, ethical AI deployment, and regulatory compliance.
Success in financial services today isn’t just about going digital—it’s about becoming intelligent.
X. Concluding Thoughts
Generative AI in Finance is no longer a futuristic concept—it’s a present-day strategic necessity. From driving hyper-personalisation to optimising operations and enhancing risk management, the fusion of AI and FinTech is redefining the way financial services operate and innovate.
However, successful integration demands more than technology adoption; it calls for visionary leadership, ethical implementation, and continuous adaptability to regulatory landscapes.
Organisations that embrace this transformative wave now, armed with the right strategies and trusted partners, will be the ones leading the financial ecosystems of tomorrow.
The future of FinTech and AI is here, and its potential is limitless. Partnering with a trusted Generative AI company will be key to navigating this transformation and seizing opportunities in the evolving financial landscape.
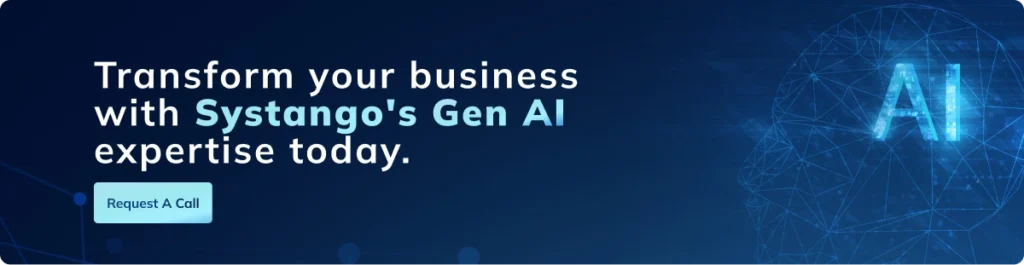
Some of the most valuable Generative AI applications in financial services include automated report generation, synthetic data creation for model training, and the generation of personalised financial insights. These innovations are transforming customer engagement, risk modelling, and compliance reporting—enabling financial institutions to operate with greater efficiency and insight.
Implementing generative AI in banking operations requires a phased approach: begin with low-risk areas like customer support automation or document generation, then expand to more complex functions like fraud detection or credit analysis. It’s essential to align AI initiatives with business goals, ensure regulatory compliance, and train staff for successful adoption.
Some promising use cases include simulating market scenarios, generating investment strategies, and producing automated portfolio insights. These tools help analysts process large datasets and make faster, more data-informed investment decisions.
Generative AI for financial risk assessment helps firms model various risk scenarios and stress-test portfolios using synthetic data. It enhances predictive analytics by generating potential market events, improving the accuracy of credit scoring and enabling pre-emptive risk mitigation strategies.
The best practices include starting with a clear use case, selecting scalable models, prioritising ethical and transparent AI usage, and embedding robust data governance. It’s also crucial for startups to choose AI tools that align with regulatory expectations from the outset.
Generative AI is highly effective because it can simulate fraudulent behaviours to train models and identify complex patterns in real-time. Enhancing anomaly detection, strengthens a bank’s ability to respond to evolving fraud techniques quickly and accurately.
Key regulatory considerations include data privacy, explainability of AI decisions, fairness in automated processes, and ongoing model validation. Financial institutions must stay updated with regional and international AI guidelines to avoid non-compliance penalties and build stakeholder trust.
Yes, typically reveals that upfront investment in AI infrastructure, training, and compliance pays off in the long term through reduced operational costs, enhanced decision-making, and increased customer satisfaction, ultimately boosting ROI.
AI-driven personalised financial advisory services use generative models to analyse individual customer data, behaviours, and goals. This enables financial institutions to deliver tailored investment plans, savings strategies, and risk insights, making advice more relevant, scalable, and efficient.
The main include data silos, outdated infrastructure, integration complexity, and limited in-house AI expertise. Overcoming these barriers requires a strategic modernisation roadmap and the use of APIs or middleware for smoother AI deployment.